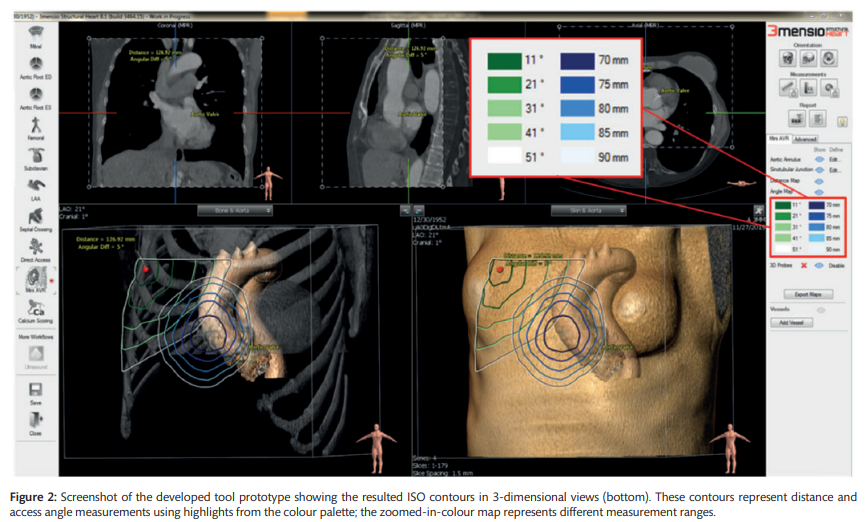
A computed tomography-based planning tool for predicting difficulty of minimally invasive aortic valve replacement
OBJECTIVES Minimally invasive aortic valve replacement has proven its value over the last decade by its significant advancement and reduction in mortality, morbidity and admission time. However, minimally invasive aortic valve replacement is associated with some on-site difficulties such as limited aortic annulus exposure. Currently, computed tomography scans are used to evaluate the anatomical relationship among the intercostal spaces, ascending aorta and aortic valve prior to surgery. We hypothesized that quantitative measurements of access distance and access angle are associated with
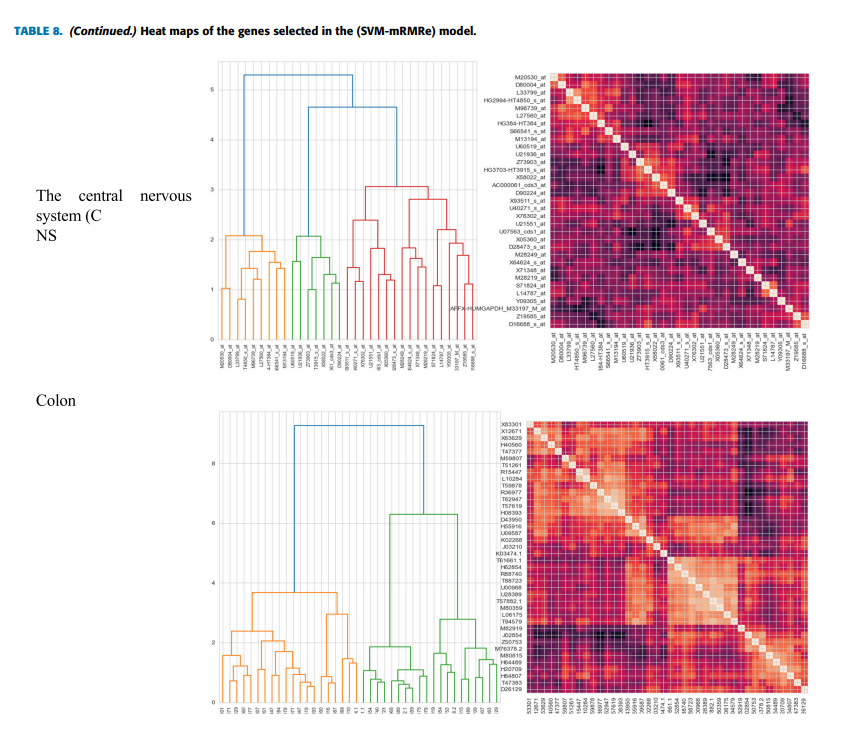
An Efficient SVM-Based Feature Selection Model for Cancer Classification Using High-Dimensional Microarray Data
Feature selection is critical in analyzing microarray data, which has many features (genes) or dimensions. However, with only a few samples the large search space and time consumed during their selection make selecting relevant and informative genes that improve classification performance a complex task. This paper proposed a hybrid model for gene selection known as (SVM-mRMRe), the proposed model provides a framework for combining filter-based, ensemble, and embedded methods to select the most relevant and informative genes from high-dimensional microarray data by fusing embedded SVM
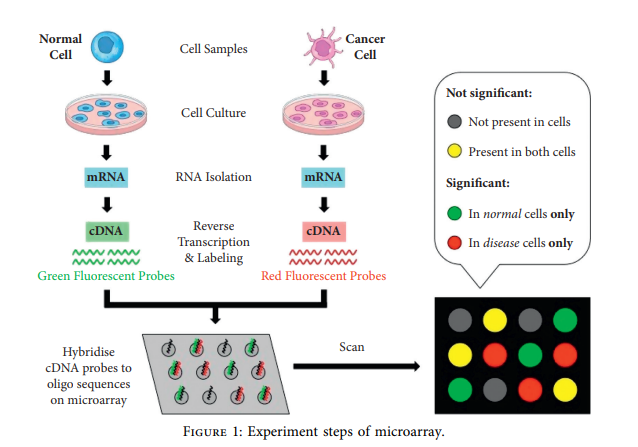
An Efficient Cancer Classification Model Using Microarray and High-Dimensional Data
Cancer can be considered as one of the leading causes of death widely. One of the most effective tools to be able to handle cancer diagnosis, prognosis, and treatment is by using expression profiling technique which is based on microarray gene. For each data point (sample), gene data expression usually receives tens of thousands of genes. As a result, this data is large-scale, high-dimensional, and highly redundant. The classification of gene expression profiles is considered to be a (NP)-Hard problem. Feature (gene) selection is one of the most effective methods to handle this problem. A
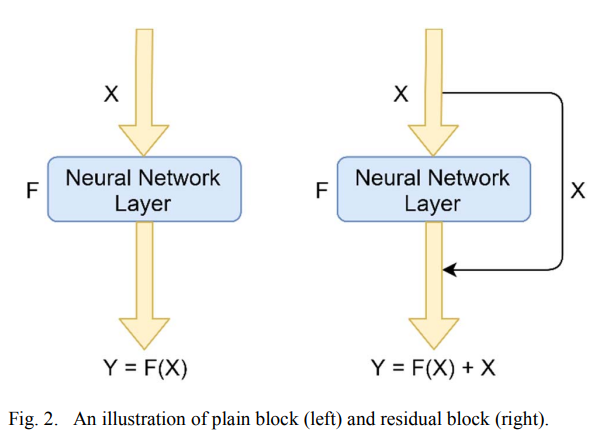
Deep Ensemble Learning for Skin Lesion Classification from Dermoscopic Images
Skin cancer is one of the leading causes of death globally. Early diagnosis of skin lesion significantly increases the prevalence of recovery. Automatic classification of the skin lesion is a challenging task to provide clinicians with the ability to differentiate between different kind of lesion categories and recommend the suitable treatment. Recently, Deep Convolutional Neural Networks have achieved tremendous success in many machine learning applications and have shown an outstanding performance in various computer-assisted diagnosis applications. Our goal is to develop an automated
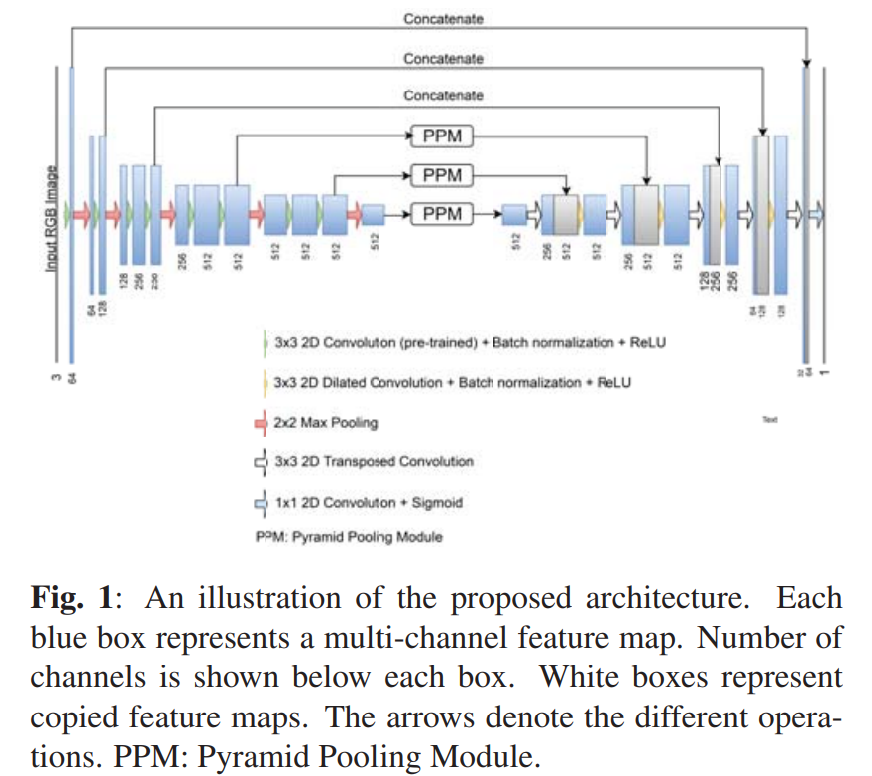
Deep convolutional encoder-decoders with aggregated multi-resolution skip connections for skin lesion segmentation
The prevalence of skin melanoma is rapidly increasing as well as the recorded death cases of its patients. Automatic image segmentation tools play an important role in providing standardized computer-assisted analysis for skin melanoma patients. Current state-of-the-art segmentation methods are based on fully convolutional neural networks, which utilize an encoder-decoder approach. However, these methods produce coarse segmentation masks due to the loss of location information during the encoding layers. Inspired by Pyramid Scene Parsing Network (PSP-Net), we propose an encoder-decoder model
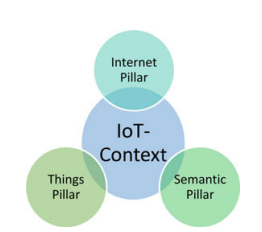
Security and Interoperability Issues with Internet of Things (IoT) in Healthcare Industry: A Survey
Recently, public healthcare systems become one of the most pivotal parts in our daily life. Resulting in an insane increase in Medical data like medical images and patient information. Having huge amount of data requires more computational power for efficient data management. In addition, data security, privacy and trustworthy have to be maintained and guaranteed. Most medical information in the last years aggregated data from a lot of devices, smart chips, tiny sensors and wearable devices. Those devices are connected through the internet, thus called Internet of Things (IoT). These devices
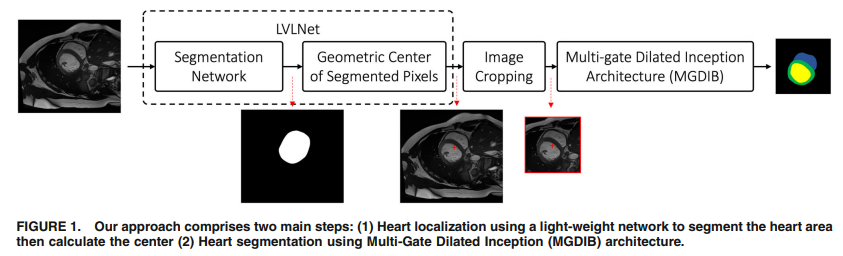
Light-Weight Localization and Scale-Independent Multi-gate UNET Segmentation of Left and Right Ventricles in MRI Images
Purpose: Heart segmentation in cardiac magnetic resonance images is heavily used during the assessment of left ventricle global function. Automation of the segmentation is crucial to standardize the analysis. This study aims at developing a CNN-based framework to aid the clinical measurements of the left ventricle and right ventricle in cardiac magnetic resonance images. Methods: We propose a fully automated framework for localization and segmentation of the left ventricle and right ventricle in both short- and long-axis views from cardiac magnetic resonance images. The localization module
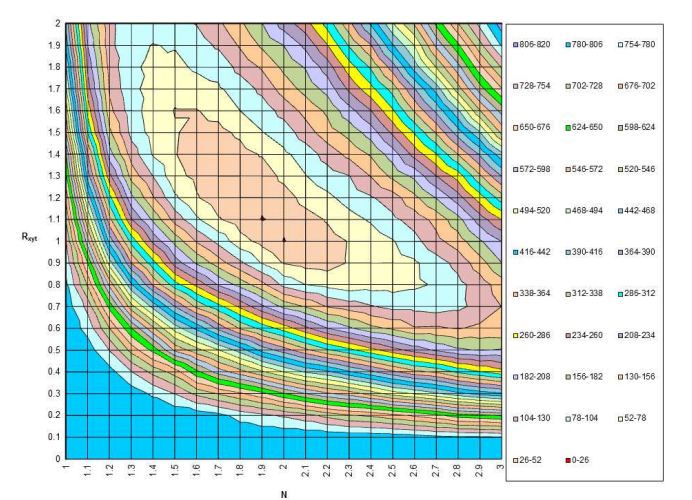
Automated classification technique for edge-on galaxies based on mathematical treatment of brightness data
Classification of edge-on galaxies is important to astronomical studies due to our Milky Way galaxy being an edge-on galaxy. Edge-on galaxies pose a problem to classification due to their less overall brightness levels and smaller numbers of pixels. In the current work, a novel technique for the classification of edge-on galaxies has been developed. This technique is based on the mathematical treatment of galaxy brightness data from their images. A special treatment for galaxies' brightness data is developed to enhance faint galaxies and eliminate adverse effects of high brightness backgrounds
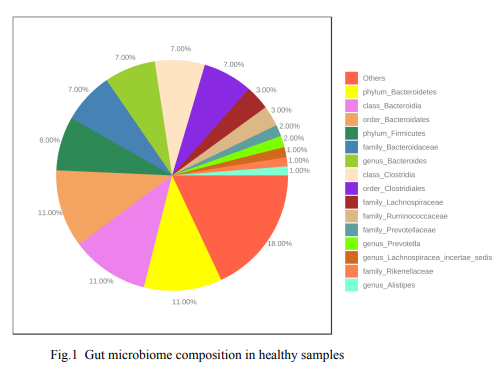
Potential probiotics for viral triggered type 2 diabetes
The scientific literature is full of studies that provide evidence highlighting the role of microbiome in type 2 diabetes (T2D) development and progression, still, discrepancies are evident when studying the link between certain taxonomic groupings and T2D, thus, eliminating the discrepancy between such studies is crucial to build on a robust systematic approach to identify the possible linkage between such taxonomic groups and diabetes development and progression, hence developing a potential treatment. Here we aimed to use a publicly available data set of gut and nares microbiome of
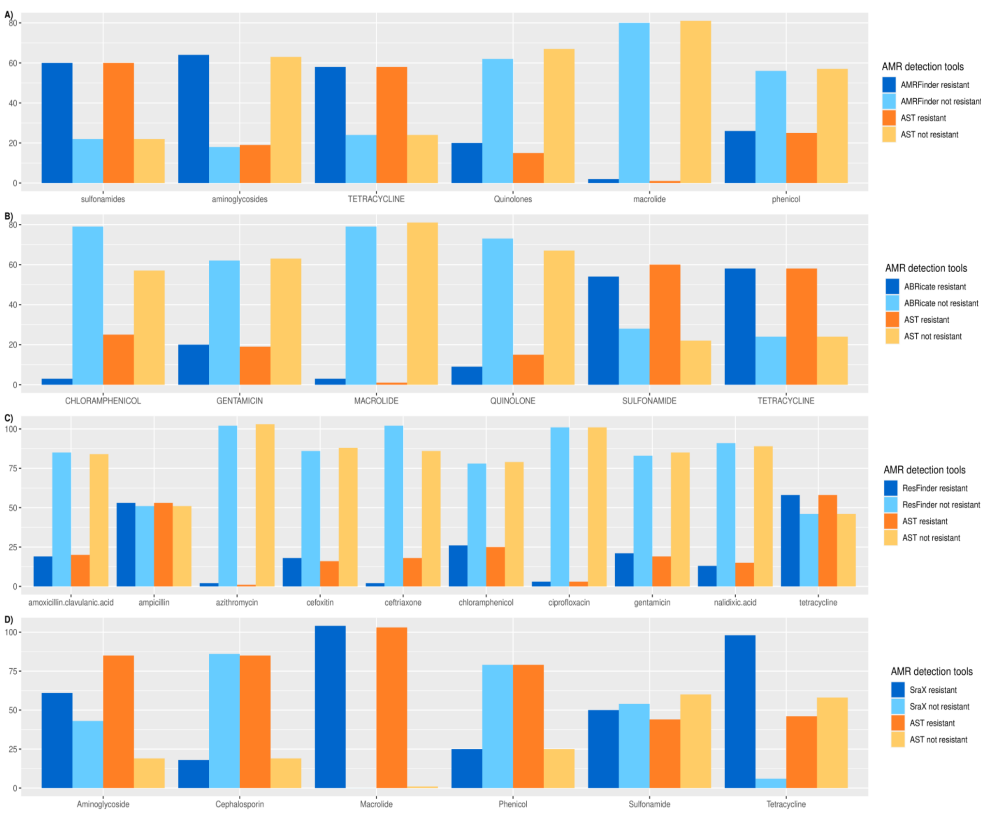
Benchmarking of Antimicrobial Resistance Gene Detection Tools in Assembled Bacterial Whole Genomes
Antimicrobial resistance (AMR) is one of the ten dangers threatening our world, according to the world health organization (WHO). Nowadays, there are plenty of electronic microbial genomics and metagenomics data records that represent host-associated microbiomes. These data introduce new insights and a comprehensive understanding of the current antibiotic resistance threats and the upcoming resistance outbreak. Many bioinformatics tools have been developed to detect the AMR genes based on different annotated databases of bacterial whole genome sequences (WGS). The number and structure of
Pagination
- Previous page ‹‹
- Page 16
- Next page ››