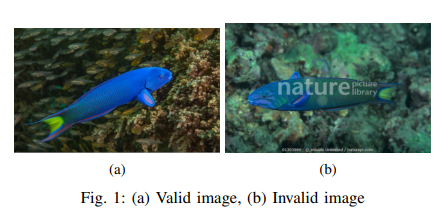
Real-Time Fish Detection Approach on Self-Built Dataset Based on YOLOv3
Creating a model to detect freely moving fish underwater in real-time is a challenging process for two main reasons. First, the available datasets suffer from some limitations that severely affect the results of the detection models operating in challenging and blurry environments. These models should be able to capture all of the fish movement given different types of surroundings. Second, choosing the convenient detection model system which matches the desired requirements from having high accuracy with satisfying frames per second (FPS). To overcome the first challenge, a new dataset was created by extracting 1800 frames from videos and manually annotating them to overcome the different background issues and the complex movements and orientations of the fish. Regarding the second challenge and after comparing between different object detection systems, YOLOv3 was chosen as it proved to achieve high accuracy among other systems. The proposed approach scored 76.81% using (mean average precision) mAP as an accuracy metric and 89.17% using F-score, which is considered one of the most accurate outcomes among the literature. Moreover, the model rate is 12 FPS which is satisfying for real-time. © 2021 IEEE.