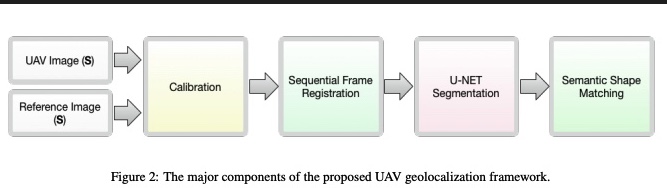
A deep CNN-based framework for enhanced aerial imagery registration with applications to UAV geolocalization
In this paper we present a novel framework for geolocalizing Unmanned Aerial Vehicles (UAVs) using only their onboard camera. The framework exploits the abundance of satellite imagery, along with established computer vision and deep learning methods, to locate the UAV in a satellite imagery map. It utilizes the contextual information extracted from the scene to attain increased geolocalization accuracy and enable navigation without the use of a Global Positioning System (GPS), which is advantageous in GPS-denied environments and provides additional enhancement to existing GPS-based systems. The framework inputs two images at a time, one captured using a UAV-mounted downlooking camera, and the other synthetically generated from the satellite map based on the UAV location within the map. Local features are extracted and used to register both images, a process that is performed recurrently to relate UAV motion to its actual map position, hence performing preliminary localization. A semantic shape matching algorithm is subsequently applied to extract and match meaningful shape information from both images, and use this information to improve localization accuracy. The framework is evaluated on two different datasets representing different geographical regions. Obtained results demonstrate the viability of proposed method and that the utilization of visual information can offer a promising approach for unconstrained UAV navigation and enable the aerial platform to be self-aware of its surroundings thus opening up new application domains or enhancing existing ones. © 2018 IEEE.