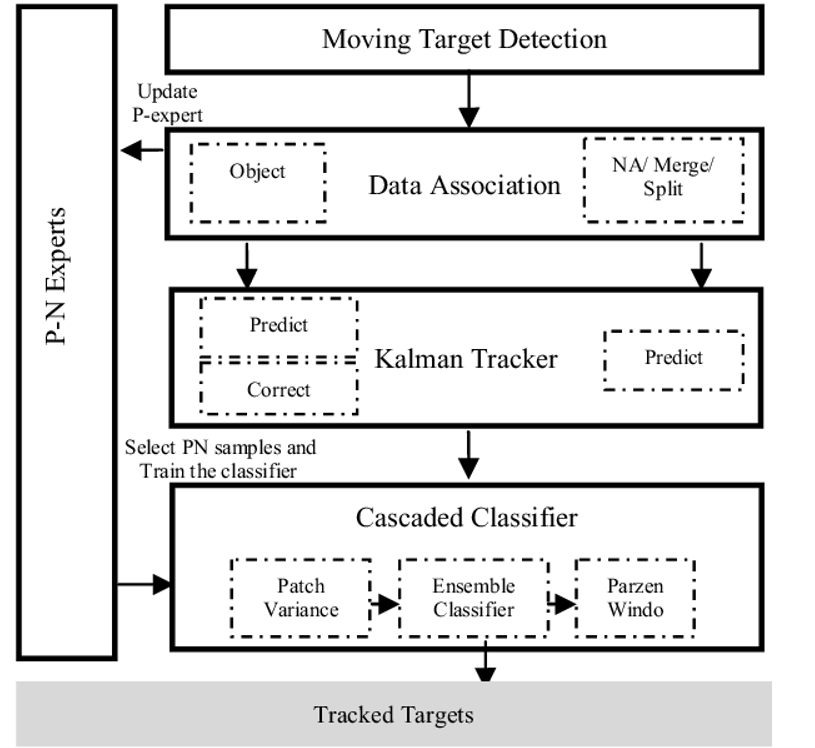
Enhanced target tracking in UAV imagery with P-N learning and structural constraints
This paper presents improved automatic moving target detection and tracking framework that is suitable for UAV imagery. The framework is comprised of motion compensation phase to detect moving targets from a moving camera, target state estimation with Kalman filter, and overlap-rate-based data association. Finally, P-N learning is used to maintain target appearance by utilizing novel structural constraints to select positive and negative samples, where data association decisions are used as positive (P) constraints. After learning target appearance, a cascaded classifier is employed to detect the target in case of association failure. The proposed framework enables to recapture targets after being out of camera field of view and helps discriminating between targets with similar appearance while alleviating drift problems. Experimental results obtained with publicly available DARPA aerial datasets demonstrate that the proposed tracker with automatic detection feedback achieves better recall and average overlap than existing manually-initialized trackers. © 2013 IEEE.