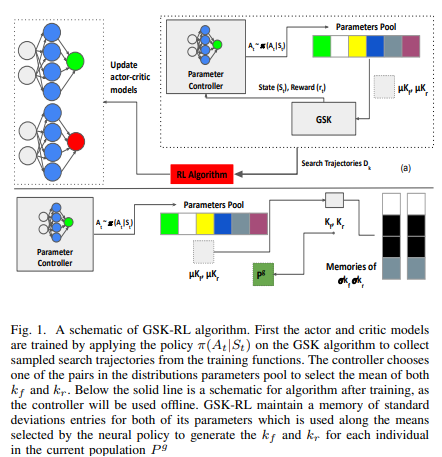
GSK-RL: Adaptive Gaining-sharing Knowledge algorithm using Reinforcement Learning
Meta-heuristics and nature inspired algorithms have been prominent solvers for highly complex, nonlinear and hard optimization problems. The Gaining-Sharing Knowledge algorithm (GSK) is a recently proposed nature-inspired algorithm, inspired by human and their tendency towards growth and gaining and sharing knowledge with others. The GSK algorithm have been applied to different optimization problems and proved robustness compared to other nature-inspired algorithms. The GSK algorithm has two main control parameters kfand kr which controls how much individuals gain and share knowledge with their common society and circles or what they inherit from their parents. GSK has no control parameter adaptation scheme, the kf and kr are fixed for all individuals. In this paper we introduce an adaptation technique for the GSK algorithm by learning those parameters during search procedure of the algorithm. The new algorithm is dubbed as GSK-RL. The parameter controller in GSK-RL is a neural network trained using actor critic methods for reinforcement learning. The GSK-RL is compared against original GSK algorithm with its default parameters on CEC 2017 test suite functions for dimensions 10 and 30. The GSK-RL performed well on 10 dimensional problems but the performance started to degrade on 30 dimensional problems and it showed unstable behaviour on some functions that the controller has never been trained on before. This paper concludes that neither the state of the search algorithm as described for the RL model nor the reward function has a critical role in designing an RL-based controller for parameters of the GSK, however the training functions and the collected trajectories are the most important factor in designing such a controller. © 2021 IEEE.